Demystifying Data Analytics: A Beginner’s Guide to Understanding the Basics
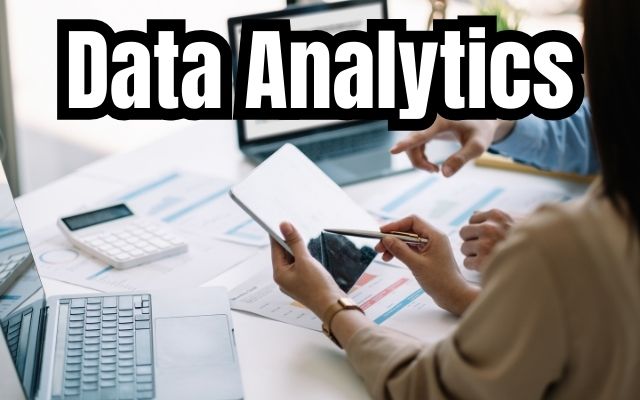
Demystifying Data Analytics is the first step towards understanding the vast world of data. In today’s digital age, data is everywhere. It’s in the apps we use, the websites we visit, and even in the devices we use. But what does all this data mean? How can we make sense of it?
This is where data analytics comes in. Data analytics is the science of analyzing raw data to make conclusions about that information. It involves many techniques with many different goals. The insights from data are used to guide strategic decision-making across industries. This guide will take you through the basics of this fascinating field.
In the dynamic landscape of contemporary digital age, records analytics has emerged as a pivotal device for groups and agencies in search of actionable insights. However, for beginners, delving into information analytics can take effort and time. This article targets to demystify the basics of statistics analytics, supplying a clear and concise guide for the ones taking their first steps into this fascinating area.
Understanding Data Analytics:
Data is the cornerstone of all analytics. It’s the raw information that we use to draw conclusions, make predictions, and drive decision-making. But what exactly is data?
In its simplest form, data is a collection of facts, statistics, or information that are represented in various forms. It can be numbers, text, images, audio, and more. Data can be classified into two main types: structured and unstructured.
Structured data is highly organized and formatted in a way that it’s easily searchable by data analysis tools. Examples of structured data include spreadsheets, where information is organized in rows and columns, or databases, where data is stored in tables.
On the other hand, unstructured data is not organized in a pre-defined manner or does not have a pre-defined data model, making it less straightforward to analyze. This includes data like text, social media posts, videos, customer reviews, etc.
At its middle, facts analytics includes examining uncooked information to attract significant conclusions and make informed selections. The technique typically encompasses records series, cleaning, analysis, and interpretation, leading to the extraction of valuable insights. Let’s spoil down the essential records analytics additives to make the journey more understandable.
What is Data Analytics?.
Data Analytics is a process that involves inspecting, cleansing, transforming, and modeling data with the goal of discovering useful information, drawing conclusions, and supporting decision-making. It is a multidisciplinary field that uses scientific methods, processes, algorithms, and systems to extract knowledge and insights from structured and unstructured data.
Data analytics can be broken down into four primary types, each serving a different purpose and providing different insights:
- Descriptive Analytics: This type of analytics answers the question, “What happened?” It involves analyzing historical data to understand changes over time.
- Diagnostic Analytics: Diagnostic analytics goes a step further to answer, “Why did it happen?” It involves more detailed data exploration and drill-down techniques.
- Predictive Analytics: Predictive analytics uses statistical models and forecasts techniques to understand the future. It answers the question, “What could happen?”
- Prescriptive Analytics: The most advanced type of data analytics. It uses optimization and simulation algorithms to advise on possible outcomes. It answers the question, “What should we do?”
Understanding these types of data analytics can help businesses and individuals make more informed decisions, predict trends, and improve operational efficiency. In the next section, we will explore various tools used in data analytics.
Data Collection:
The basis of any statistics analytics enterprise lies inside the records itself. Sources can vary from conventional databases to contemporary streams like social media, sensors, or web scraping. Understanding the sorts and formats of facts is essential for realistic analysis.
Data Cleaning:
Data is hardly ever pristine; it regularly contains errors, inconsistencies, or lacking values. Data cleansing, or information cleaning, is rectifying these issues to make certain the accuracy and reliability of the analytics method. With clean records, any subsequent analysis can be included.
Data Analysis:
This is where the magic occurs. In the evaluation segment, various statistical and mathematical strategies are employed to discover facts styles, traits, and relationships. Visualization equipment like charts and graphs are often used to make the insights greater available.
Data Interpretation:
Once the analysis is whole, it is time to interpret the findings. What do the styles and trends reveal about the commercial enterprise or hassle? This step requires analytical skills and area expertise to attract meaningful conclusions.
The Role of Database Support:
In information analytics, database support is critical in facilitating green information control. Databases are based repositories that save, prepare, and retrieve information, providing a strong foundation for analytics. A well-designed database supports the seamless storage and retrieval of extensive datasets, contributing to the general effectiveness of the analytics technique.
Types of Data Analytics.
Data analytics is a broad field with various types, each serving a different purpose and providing different insights. Here are the four primary types of data analytics:
- Descriptive Analytics: This is the most basic form of data analytics. It involves analyzing historical data to understand what has happened in the past. Techniques used include data aggregation and data mining.
- Diagnostic Analytics: This type of analytics dives deeper into data to understand the root cause of a particular outcome. It involves techniques such as drill-down, data discovery, correlations, and data mining.
- Predictive Analytics: As the name suggests, predictive analytics is used to predict future outcomes based on historical data. It uses statistical models and forecasting techniques.
- Prescriptive Analytics: This is the most advanced type of data analytics. It not only predicts future outcomes but also suggests actions to benefit from the predictions. It uses techniques like machine learning, algorithms, and computational modelling procedures.
Each type of data analytics serves a unique purpose and is used based on the specific needs of a business or project. Understanding these types can help you choose the right approach for your data analysis needs.
Data Analytics Tools:
In the world of data analytics, various tools are used to analyze and interpret data. Here are some of the most commonly used ones:
- Excel: Microsoft Excel is one of the most basic and widely used data analysis tools. It offers functionalities like spreadsheet capabilities, data visualization, and simple statistical functions.
- Python: Python is a high-level programming language that is widely used in data analysis. It has libraries like Pandas, NumPy, and Matplotlib that make data analysis and visualization easier.
- R: R is a programming language and software environment specifically designed for statistical computing and graphics. It is widely used for data manipulation, data analysis, and visualization.
- SQL: SQL (Structured Query Language) is used to manage and manipulate databases. Data analysts use SQL to query, insert, update, and modify data.
- Tableau: Tableau is a data visualization tool that is widely used in the industry. It allows data analysts to create interactive dashboards and reports.
- Power BI: Power BI is a business analytics tool developed by Microsoft. It provides interactive visualizations and business intelligence capabilities with an interface that is easy to use for creating reports and dashboards.
- SAS: SAS (Statistical Analysis System) is a software suite developed by SAS Institute for advanced analytics, multivariate analyses, business intelligence, data management, and predictive analytics.
- Apache Hadoop: Apache Hadoop is an open-source software framework used for distributed storage and processing of dataset of big data.
Each tool has its own strengths and is used based on the specific needs of the project. Understanding these tools can help you choose the right one for your data analysis needs.
Applications of Data Analytics:
Data analytics is a powerful tool that has applications in various fields. Here are some of the key areas where data analytics is making a significant impact:
- Business: Businesses use data analytics for market research, customer segmentation, and decision making. It helps businesses understand their customers better, improve their products and services, and make informed decisions.
- Healthcare: In healthcare, data analytics is used for disease surveillance, patient care, and research. It helps in predicting disease outbreaks, improving patient care, and discovering new medical insights.
- Finance: In finance, data analytics is used for risk assessment, fraud detection, and investment decisions. It helps financial institutions manage risks, detect fraudulent activities, and make profitable investments.
- Sports: In sports, data analytics is used for player performance analysis, team strategy, and injury prevention. It helps teams and coaches understand player performance, devise effective strategies, and prevent injuries.
- Education: In education, data analytics is used for student performance analysis, curriculum development, and resource allocation. It helps educational institutions understand student performance, develop effective curriculums, and allocate resources efficiently.
- Government: In government, data analytics is used for policy making, public service delivery, and crime prevention. It helps governments make informed policies, deliver public services effectively, and prevent crimes.
These are just a few examples of how data analytics is being used across different fields. The possibilities are endless and the impact is significant.
Conclusion: Demystifying Data Analytics
Demystifying records analytics for novices includes unraveling the complexity of facts-related methods. Each step performs a critical function in extracting valuable insights from collection to interpretation.
Understanding the significance of database assist on this adventure is crucial, as it forms the backbone of efficient information control. Armed with these fundamentals, novices can confidently embark on their records analytics adventure, geared up to discover the enormous possibilities this discipline gives.